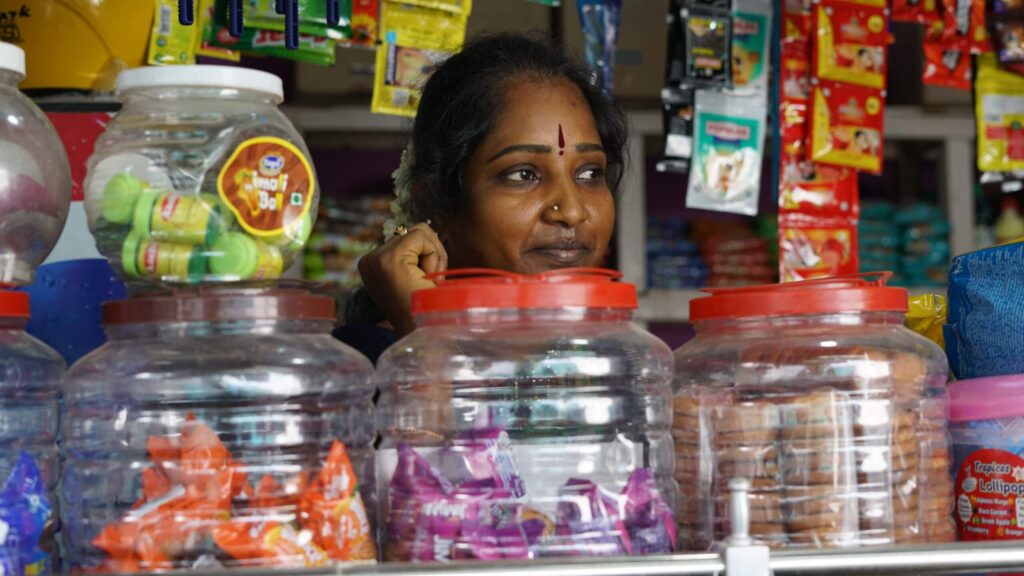
From insurance to mobile payments, many of us often take for granted the financial services that make our lives easier. But, for nearly two billion underserved people around the world, these tools are out of reach.
At the same time, we are seeing an explosion of new sources of data, and our ability to analyze data and see patterns. Phone usage, school tuition, utility payments, satellite images — all can be used to create a clear picture of an individual or her small business, as a basis for providing responsible financial services.
But algorithms may exacerbate existing disparities. If biased, they can easily exclude instead of include, especially underserved people with nascent data footprints.
Impact investors who find, shape, and support artificial intelligence (AI) innovations have a major role to play in advancing responsible and equitable AI. That’s why, with support from USAID, the Center for Financial Inclusion at Accion has just published an equitable AI toolkit for investors or anyone involved in the development of AI-based tools.
Think of this as “AI hygiene,” or questions that leaders should ask before backing a new solution. Does your data include those who are typically underrepresented? Are your outcomes fair? Does your team reflect the demographics of your user base? Are they trained on algorithmic bias?
Making the invisible, visible
It is increasingly important that investors and fintech leaders, who are on the frontier of this innovation start asking these questions. Their work is already showing the power of AI for inclusion. Take these examples from companies in Accion Venture Lab’s portfolio:
In Kenya, Apollo Agriculture is using predictive algorithms to analyze satellite data to provide crop insurance and financing for small farms. Apollo combines this with data-driven advice and agricultural inputs to help farmers boost their crop yields. Their work is increasingly vital as low-income farmers confront the most severe effects of climate change.
And Field Intelligence is using AI to simplify the medical supply chain across Africa. Using AI, Field guides local pharmacies on precisely which drugs to stock. Field’s network of more than 2,000 small pharmacies allows them to recognize patterns that an individual store owner could never see. And with Field’s financing, clients essentially only pay for what they can sell, keeping their communities healthy and improving their revenues. AI is the key.
And AI has the potential to revolutionize customer service. Underserved clients often aren’t comfortable using financial apps, and when they do, they often need human support. Now, with generative AI, we can replicate or augment person-to-person interactions, and in native languages to create deeper client engagement – and trust.
Addressing algorithmic bias
If used well, AI also has the power to reduce human bias that has long undermined financial access. One U.S. study found that fintech algorithms discriminated 40 percent less on average than loan officers in loan prices.
Yet, just like the people who use and design them, algorithms can be biased. When they are, their impacts extend far beyond that of one biased employee. One flawed algorithm used by a U.S. healthcare company affected more than 200 million people annually, systematically recommending white patients for more comprehensive care than equally sick black patients.
Bias can creep into algorithms at any stage of their development. Even if developers get it all right, algorithms can produce fair results one day, then evolve to become biased later.
For example, many fintech companies in emerging markets leverage phone data to assess clients’ creditworthiness. If you regularly recharge and top up your phone, presumably, you are more likely to repay a loan. But digging deeper reveals that men in these markets typically have their own phone, while women use the family phone, which inevitably runs out of power and minutes. Today, we are designing algorithms that systematically discriminate against women. We need to do better.
Further, the data footprints of low-income people may make them appear riskier in the eyes of an algorithm. For example, since Black and Latino borrowers in the U.S. have been historically victimized by predatory lenders, their historical data can systematically lead to biased algorithmic decisions.
“AI hygiene” supports inclusive progress
We cannot continue to treat algorithms as if they are a black box. And we cannot settle for “fairness through unawareness,” when algorithms only appear to be fair, simply because we lack a full picture of those affected. Taking a closer look at algorithms’ outcomes requires responsibly collecting sensitive demographic data and working with regulators to ensure this is done appropriately.
The EU AI Act is one example of a significant step in the right direction. The proposed law takes a bold stance on greater transparency and the use of biometric data, and while there has been pushback from the private sector, collaboration and flexibility can accommodate both rapid innovation and strong consumer protections. More are needed.
The potential benefits of AI for inclusion are enormous, but to truly realize them, we must fully understand and answer the risks. As we develop these powerful tools, the future is very bright, especially if we can identify and minimize the blind spots.